Topic: Operations
In memoriam: George Strauss, 1923-2020, an icon in the field of industrial relations
4 years after acquisition by Emmi, founders of Sonoma County’s Cowgirl Creamery retire
To catch the most COVID-19 cases, testing policies should vary based on demand, study finds
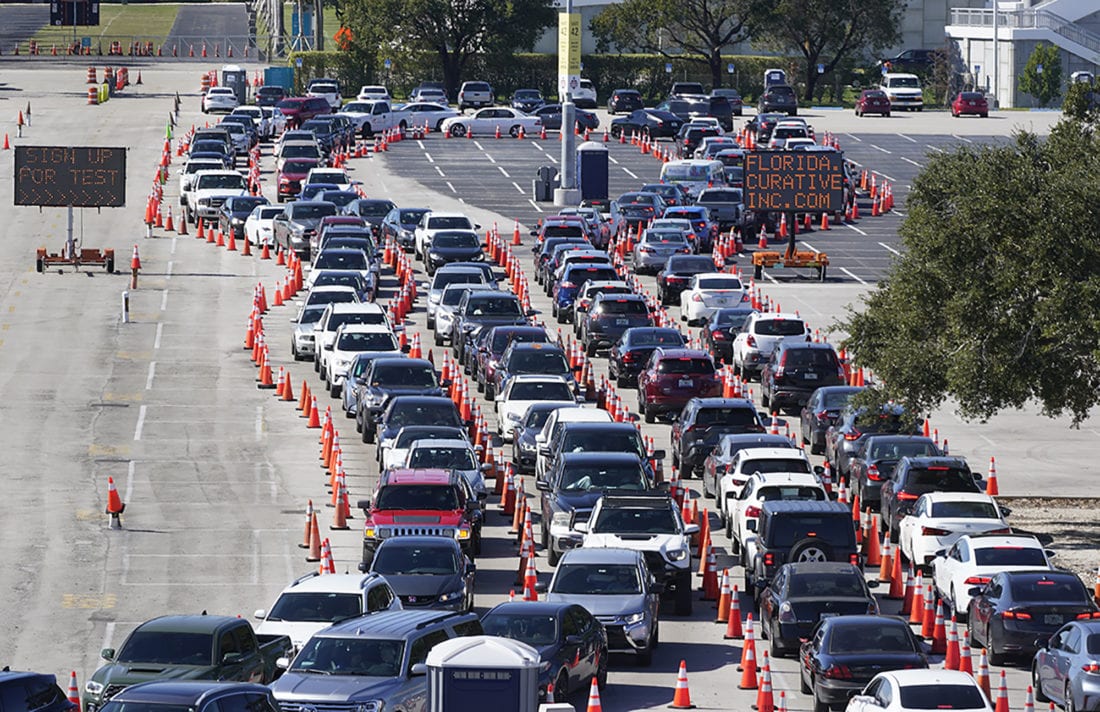
With months to go in the mass rollout of COVID-19 vaccines, testing is still critical to control the surging pandemic. Yet with testing capacity still limited in some areas, health authorities have struggled with how to catch the most cases: Test on a first-come, first-served basis, or prioritize testing for those with symptoms? Provide free testing for all?
In a recent working paper, Berkeley Haas Asst. Prof. Luyi Yang found that free testing for all—with no prioritization—can backfire when demand gets too high. Along with co-authors Shiliang Cui and Zhongbin Wang, Yang developed a model to project how testing facilities should schedule and price COVID testing to reduce infection rates.
“Long lines may discourage people from getting tested promptly, which of course can be dangerous from a public health standpoint,” Yang says. “My co-authors and I figured there had to be a way to make testing lines more efficient.”
Long lines may discourage people from getting tested promptly, which of course can be dangerous from a public health standpoint.
Testing centers in some cities, like San Francisco or Washington DC, have chosen to give people equal priority regardless of whether they have any symptoms. Other agencies, like the French government, have given priority to symptomatic people.
Yang and his co-authors’ model predicted that free testing without prioritizing those more at risk does not identify the most cases if testing demand is high. That’s because when testing facilities become overly congested, free testing results in too many asymptomatic people getting tested at the expense of symptomatic people, who are most likely to carry the virus. They concluded that when demand is higher than capacity, charging a fee increases the likelihood of testing more symptomatic people, because asymptomatic people’s decisions are more sensitive to price.
Conversely, they found that if a priority testing policy is used, testing should be free. When people with symptoms are given priority, the decisions of asymptomatic people are no longer a factor in their decision whether to get tested—even if lines are long, they’ll get to move to the front. As a result, charging a fee will only discourage both symptomatic and asymptomatic people from getting tested. This policy is exactly what the French government does: Testing is free for all, even those with no symptoms, but symptomatic or high-risk people are given priority.
Policies to detect the most cases
This analysis raised the question of which approach testing facilities should take. Yang said that the most important move facilities should make is to be flexible with their scheduling policy.
When testing demand is low and there’s no wait time, the researchers’ model predicts that either policy will be effective. As testing demand surges, priority testing for symptomatic people catches more cases.
However, when there is a moderate level of demand, the researchers’ findings suggest that it does not make sense to give priority to symptomatic people. High-risk people are still likely to get tested, but the hassle of waiting might push low-risk people away, reducing the chance of identifying asymptomatic carriers. In those situations, the best policy is in fact to prioritize people without symptoms.
“All else equal, people without symptoms are less motivated to seek a test, so if there is enough capacity, we would like to create an incentive for more of them to get tested,” Yang says.
All else equal, people without symptoms are less motivated to seek a test, so if there is enough capacity, we would like to create an incentive for more of them to get tested.
Though there are certainly other factors that determine the spread of the disease, Yang believes there are actionable items that testing facilities can take depending on the demand for testing.
“The high-level takeaway is that the optimal testing policy depends on the level of testing demand relative to testing capacity, so it should vary by region and evolve over time. That is, one should caution against a one-size-fits-all approach,” Yang says.
Facade of workers’ safety beginning to show cracks during the pandemic
MIT Sloan/Glassdoor study offers surprising findings on corporate culture
Investing in AI boosts firm growth—and increases market dominance, study finds
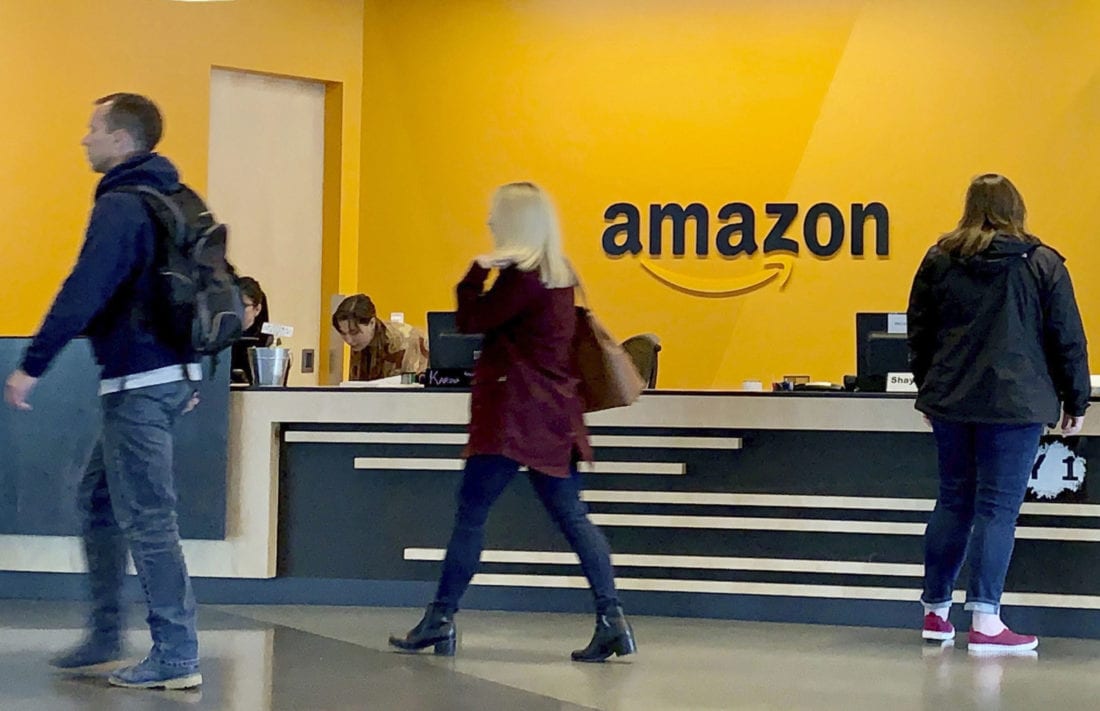
Large companies with their greater resources have a natural advantage when it comes to investing in artificial intelligence. Those investments, in turn, help those large companies to grow even larger—creating a virtuous circle that leads to even greater market dominance.
In short, AI has helped “superstar” firms such as Amazon, JPMorgan Chase, and UnitedHealth, get even bigger, according to a new paper co-authored by Berkeley Haas Assistant Professor Anastassia Fedyk.
“AI investments have been concentrated in large firms that were already productive, enabling those firms to grow even larger and capture even more market share,” Fedyk said. “By facilitating the rise of the ‘superstar’ firms, the new technology is leading to increases in industry concentration.”
Along with co-authors Tania Babina, an assistant professor at Columbia Business School; Alex He, an assistant professor at the University of Maryland; and James Hodson, the CEO of the AI for Good Foundation, Fedyk found that firms that invested more in AI technology increased their sales and employment, leading to higher levels of industry concentration.
“We wanted to look at whether this recent technological change adopted by firms led to something concrete,” Fedyk said. “Is it the case that modern technology is improving firm productivity and stimulating growth?”
The answer to that was mixed, they found.
Analyzing AI job postings and resumes
AI technologies, such as machine learning or natural language processing, rely on computer science to simplify tasks involving decision-making or forecasting. Because AI relies on big datasets, it’s possible that larger firms have an advantage because they have more data and can more efficiently tailor products to different consumers. Moreover, this streamlined technology may simplify production, resulting in higher firm productivity.
Quantifying how AI affects the economy has been difficult because there is little data on individual firms’ AI usage. Fedyk and her coauthors overcame this challenge by using online job postings and employee resume data to look at how many of the firms’ job postings or workers had AI-related skills and create an index of companies’ use of AI.
The researchers then looked at the types of companies that use AI technology. They found higher investments in AI by “superstar” firms—those with larger market shares, higher cash reserves, and higher R&D intensity. The ten firms with the most AI jobs were Amazon.com Inc., International Business Machines Corp. (IBM), Microsoft Corp., Apple Inc., Facebook Inc., Intel Corp, Alphabet Inc., Capital One Financial Corp., multinational consulting firm BearingPoint Inc., and General Electric Co. However, the rise in AI investments from 2010 to the present has been ubiquitous across all industry sectors, with roughly a sixfold increase in the share of AI workers across the board.
Growth for the largest firms
In terms of economic growth, the researchers found that companies investing more in AI technology had higher growth rates from 2010 to 2018. A one-standard-deviation increase in the share of AI workers was associated with a 16% growth in sales, and, interestingly, a 15% increase in employment. The researchers note that this last finding assuages some of the conventional concern that AI technology might destroy more jobs than it creates.
Unlike the adoption of robots, which other studies have found increases employment at the firm level but decreases employment at the industry level, Fedyk found that firm-level growth from AI investments did translate into industry-level growth in sales and employment. In other words, firms benefitting from AI did not, in general, do so at the expense of other companies within their industry.
The researchers concluded that the findings were driven by firms that were already larger and more productive before the adoption of AI. For instance, the largest third of companies increased sales by 17%, while the smallest third of companies saw no increase at all. Moreover, the large firms that adopted AI simultaneously expanded into new geographic and product markets. The researchers interpret these results as evidence that AI has helped the most productive firms scale more efficiently.
Fedyk points out that while this growth has led to greater market concentration towards the largest firms, that’s not necessarily a bad thing, as more market share is being allocated to more productive firms.
At the same time, there was no evidence that AI-adopting firms became more productive as a result of the technology. However, the researchers cautioned that productivity gains may take some time to materialize. “It’s still early on as AI hasn’t been around for that long,” Fedyk said. “It’s possible that it will take longer to see improvements in efficiency.”